In an era dominated by artificial intelligence (AI) and machine learning (ML), the accuracy and success of these technologies largely depend on a crucial component: data labeling. Data labeling, the process of tagging or classifying data to make it usable for AI models, directly influences the precision of the models' predictions and outcomes. Whether it’s recognizing images, understanding speech, or predicting future trends, the foundation of every powerful AI system is well-labeled data.
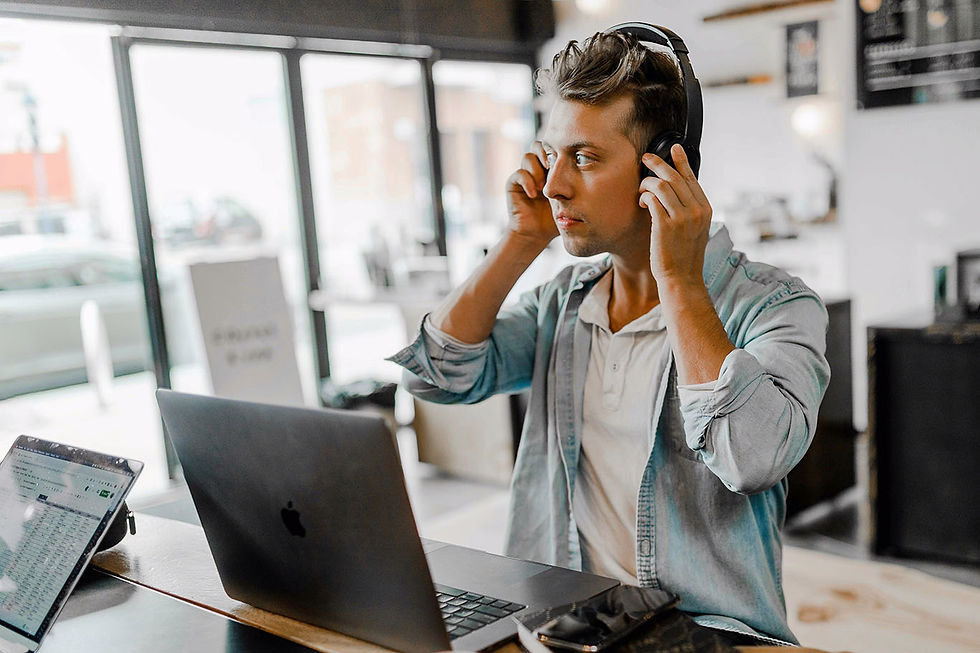
In this guide, we’ll explore essential data labeling techniques, the role they play in achieving success with AI and ML projects, and how businesses can harness the power of precision to unlock new levels of innovation and efficiency.
What Is Data Labeling?
At its core, data labeling involves annotating raw data with tags or labels to make it understandable for AI algorithms. This data can take many forms—images, videos, text, or audio—and the labels attached to it provide context that helps machine learning models interpret and learn from the data.
For example, in an image recognition task, each image might be labeled with what it depicts: a car, a dog, or a tree. Similarly, in natural language processing (NLP), text data can be labeled with information about sentiment, intent, or named entities like locations and names. These labeled datasets then serve as the training material for AI models, enabling them to "learn" patterns and make predictions when given new, unlabeled data.
The Importance of Precision in Data Labeling
The success of any AI or ML model is directly tied to the quality and precision of the labeled data it’s trained on. Incorrect or inconsistent labeling can lead to inaccurate predictions, poor model performance, and a failure to meet business objectives. Conversely, high-quality labeled data enables models to perform at their best, delivering accurate and reliable results.
Precision Drives Model Accuracy
When it comes to data labeling, precision is key. Each label needs to be accurate and consistently applied across the entire dataset. Even minor discrepancies in labeling can confuse the model and lead to errors in prediction. For instance, if a cat is mistakenly labeled as a dog in an image classification task, the model may struggle to accurately differentiate between the two in future predictions.
Ensuring that every piece of data is labeled precisely can make a significant difference in the overall accuracy of the model. As the saying goes, "Garbage in, garbage out"—if the labeled data is incorrect or inconsistent, the model’s predictions will reflect that.
Training Data Volume and Diversity
In addition to precision, the volume and diversity of labeled data also impact the success of AI and ML models. A large dataset that covers a wide range of scenarios allows the model to learn from diverse inputs and generalize better to new data. However, if the labels within this dataset are not consistently applied, the model's performance will suffer, no matter how large the dataset is.
Reducing Bias Through Consistent Labeling
Data labeling precision also plays a critical role in reducing bias in AI models. Inconsistent labeling can introduce biases that skew the model’s predictions, leading to unfair or inaccurate outcomes Business Process Outsourcing. For example, in facial recognition technology, if certain demographic groups are mislabeled or underrepresented in the training data, the model may perform poorly on those groups. By ensuring that data labeling is done consistently and accurately across all samples, businesses can reduce bias and improve the fairness of their AI systems.
Key Data Labeling Techniques for Success
Achieving precision in data labeling requires the use of effective techniques and tools. Here are some of the most widely used data labeling techniques that businesses can adopt to ensure accuracy and success in their AI and ML projects:
1. Manual Labeling
Manual labeling is the process of having human annotators review and label data by hand. This method is often necessary for complex tasks that require a deep understanding of context or subject matter expertise. For example, in medical image labeling, trained radiologists may need to annotate X-rays or MRI scans to identify signs of disease.
While manual labeling offers a high level of accuracy, it can be time-consuming and expensive, especially for large datasets. To mitigate these challenges, businesses can use a combination of manual labeling and automation to balance precision and efficiency.
2. Automated Labeling
outsourcing services uses AI and ML algorithms to label data without human intervention. While this technique is faster and more scalable than manual labeling, it may not be as accurate, especially in cases where the data is complex or ambiguous. Automated labeling is most effective when used to label large volumes of simple data, such as tagging images or classifying straightforward text.
To enhance the precision of automated labeling, many businesses use a hybrid approach that combines automation with human review. This allows for faster processing while ensuring that the labeled data meets high-quality standards.
3. Active Learning
Active learning is a machine learning technique that involves training the model with a small, labeled dataset and then allowing the model to identify which additional data points it is uncertain about. These uncertain data points are then sent to human annotators for labeling. This technique allows businesses to prioritize labeling the most valuable data points, resulting in a more efficient and targeted labeling process.
Active learning can be particularly useful for businesses working with limited resources, as it reduces the overall volume of data that needs to be labeled while still improving model accuracy.
4. Crowdsourcing
Crowdsourcing involves using a large group of people, often from online platforms, to label data. This technique can be an effective way to scale up the labeling process quickly, especially for tasks that don’t require specialized expertise. By distributing the labeling tasks across many individuals, businesses can process large datasets in a short amount of time.
However, crowdsourcing comes with its own challenges. Ensuring consistency and accuracy across multiple annotators can be difficult, and the quality of the labels may vary depending on the skill level of the contributors. To address this, businesses can implement quality control mechanisms, such as having multiple annotators label the same data point and comparing their results.
5. Semi-Supervised Learning
In semi-supervised learning, a model is trained using a small amount of labeled data and a large amount of unlabeled data. The model learns from the labeled data and applies what it has learned to label the unlabeled data. This technique allows businesses to reduce the amount of manual labeling required while still achieving a high level of accuracy.
Semi-supervised learning is particularly useful for businesses with limited labeled data but large volumes of raw, unlabeled data. By leveraging both labeled and unlabeled data, businesses can improve the performance of their AI models without the need for extensive manual labeling efforts.
Tools and Platforms for Efficient Data Labeling
The rise of AI and ML has led to the development of a wide range of data labeling tools and platforms designed to streamline the labeling process and enhance precision. These tools offer features such as automated labeling, annotation workflows, and quality control measures to ensure consistency and accuracy. Some popular data labeling platforms include:
Labelbox: A comprehensive platform that provides tools for image, video, and text annotation, along with workflow management and collaboration features.
SuperAnnotate: A platform designed for teams working on computer vision projects, offering powerful annotation tools and collaboration capabilities.
Scale AI: Known for its high-quality training data, Scale AI provides a range of annotation services, including image, text, and 3D data labeling.
Amazon SageMaker Ground Truth: A service that enables businesses to build highly accurate training datasets with the help of active learning and human annotators.
By leveraging these tools, businesses can improve the efficiency and accuracy of their data labeling processes, ensuring that their AI models are built on a strong foundation of well-labeled data.
Conclusion: Precision is the Key to AI Success
In the world of AI and machine learning, the quality of the data is everything. Accurate, consistent, and precise data labeling is essential for building AI models that deliver reliable and impactful results. By adopting effective data labeling techniques, leveraging advanced tools, and prioritizing precision, businesses can set themselves up for success in their AI and ML projects.
Whether through manual annotation, automated tools, or a hybrid approach, the key to unlocking the full potential of AI lies in the power of precision.
Comments